Annotating CryoET Volumes: A Machine Learning Challenge
Nov 4, 2024·,,,,,,,,,,,,,,,,,,·
0 min read
Ariana Peck
Yue Yu
Jonathan Schwartz
Anchi Cheng
Utz Heinrich Ermel
Saugat Kandel
Dari Kimanius
Elizabeth Montabana
Daniel Serwas
Hannah Siems
Feng Wang
Zhuowen Zhao
Shawn Zheng
Matthias Haury
David Agard
Clinton Potter
Bridget Carragher
Kyle Harrington, * Co-Corresponding
Mohammadreza Paraan, * Co-Corresponding
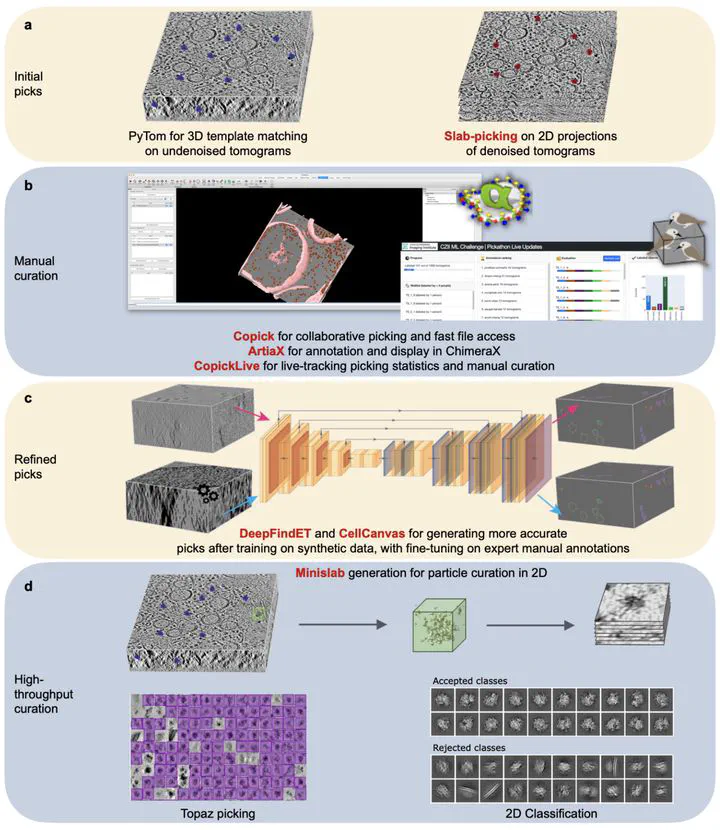
Abstract
Cryo-electron tomography (cryoET) has emerged as a powerful structural biology tool for understanding protein complexes in their native cellular environments. Presently, 3D volumes of cellular environments can be acquired in the thousands in a few days where each volume provides a rich and complex cellular landscape. Despite numerous innovations, localizing and identifying the vast majority of protein species in these volumes remains prohibitively difficult. Machine learning-based methods provide an opportunity to automate the process of labeling and annotating cryoET volumes. Due to current bottlenecks in the annotation process, and a lack of large standardized datasets, training datasets for machine learning algorithms have been scarce. Here, we present a defined “phantom” sample, along with “ground truth” annotations, that will be the basis of a machine learning challenge to bring cryoET and ML experts together and spur creativity to address this annotation problem. We have also set up a cryoET data portal that provides additional diverse sets of annotated 3D volumes from cryoET experts across the world for the machine learning challenge.
Type
Publication
bioRxiv